Machine Learning Technology: Revolutionizing the Future of Innovation
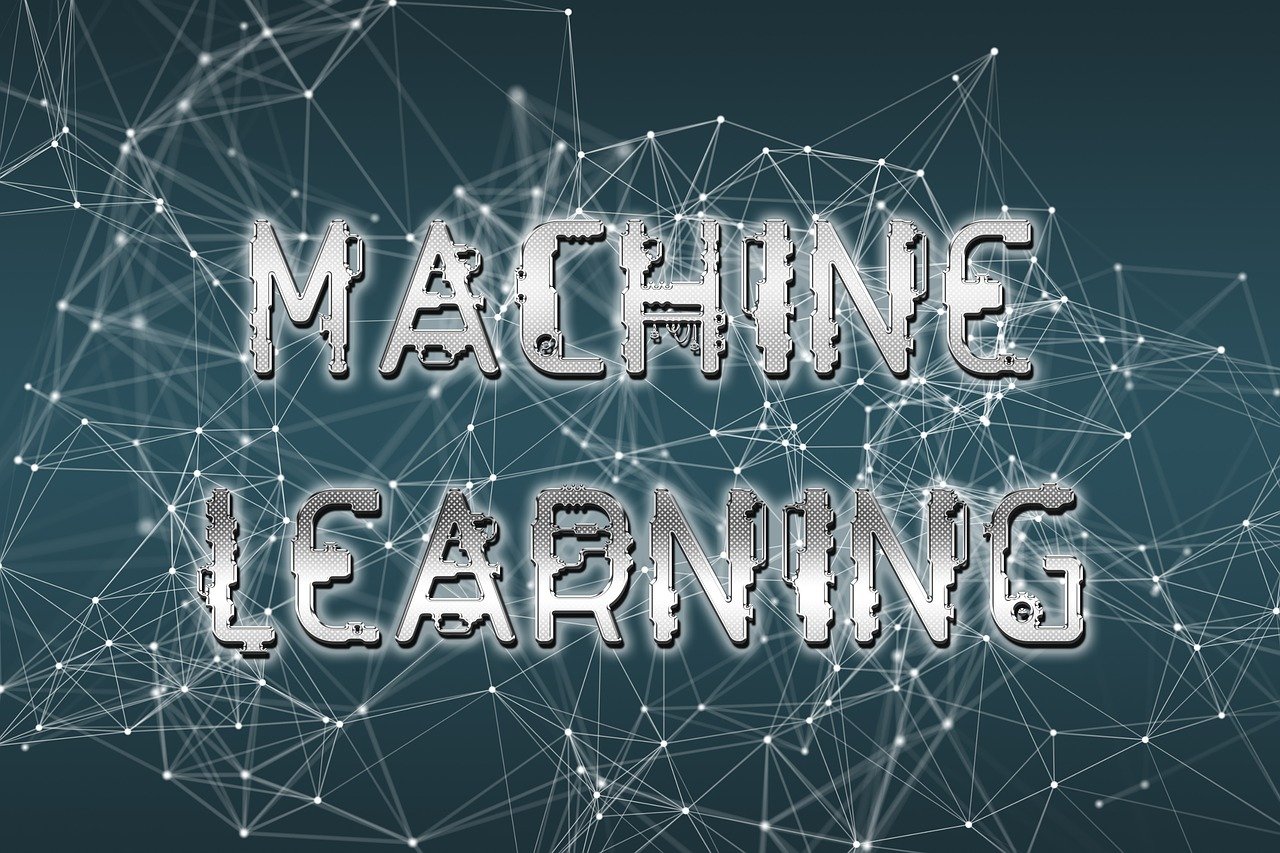
AI (ML) innovation, a subset of man-made consciousness (computer based intelligence), has arisen as perhaps of the most groundbreaking power in this day and age. With applications traversing ventures from medical services to fund, instruction, and then some, AI is reshaping the manner in which we associate with information and simply decide. By empowering machines to gain from information and pursue forecasts or choices without being expressly modified, AI is driving robotization, proficiency, and advancement.
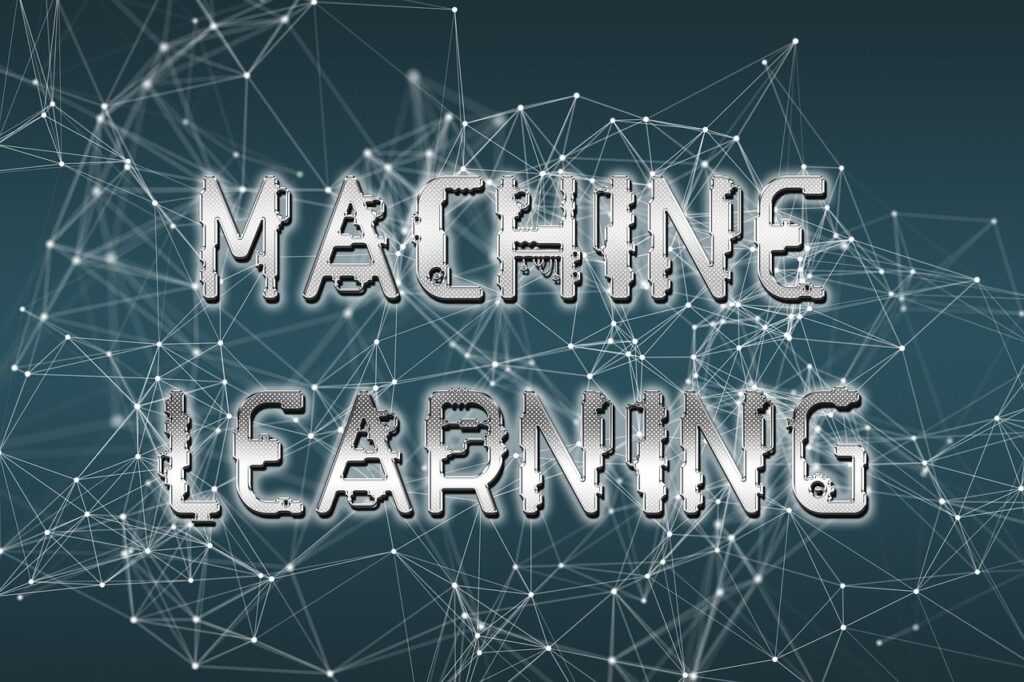
What is AI?
At its center, AI alludes to the advancement of calculations that permit PCs to gain examples and make forecasts from information. Rather than depending on pre-customized rules, an AI model works on its exhibition by breaking down enormous volumes of information, recognizing designs, and changing its methodology in light of new data.
AI is ordinarily sorted into three primary sorts:
1 Directed Realizing: This type includes preparing a model utilizing marked information (input-yield matches). The calculation learns the connection among sources of info and results, and it can anticipate the result for inconspicuous information sources.
2 Solo Learning: In unaided learning, the calculation is given unlabeled information. The objective is to recognize stowed away examples or connections inside the information, like gathering comparable things (grouping) or decreasing the dimensionality of information (head part investigation).
3 Support Learning: Here, a specialist cooperates with a climate and advances by getting prizes or punishments. The objective is to become familiar with a methodology that boosts combined compensation over the long haul. Support learning is broadly utilized in mechanical technology, gaming, and independent vehicles.
How In all actuality does AI Function?
AI models work through a progression of steps:
1 Information Assortment: The initial step is assembling huge datasets that are illustrative of the issue to be tackled. For instance, in medical care, this could include gathering patient records, while in online business, it could incorporate customer conduct information.
2 Information Preprocessing: Crude information is frequently untidy, fragmented, or boisterous. Consequently, preprocessing includes cleaning, changing, and normalizing information to guarantee its quality and reasonableness for preparing.
3 Model Choice: Different ML calculations exist, for example, choice trees, brain organizations, support vector machines, and k-closest neighbors. The decision of model relies upon the job that needs to be done.
4 Preparing: During the preparation stage, the model gains from the information by recognizing designs. It changes its inner boundaries to limit blunders in forecasts.
5 Assessment: In the wake of preparing, the model is tried on new information (approval set) to perceive how well it sums up. Assuming the presentation is unsuitable, changes are made to further develop exactness.
6 Sending: When the model is adequately exact, settling on certifiable forecasts or choices is sent. Persistent observing and retraining might be important as new information is gathered.
Utilizations of AI
AI is an unavoidable innovation with applications across numerous areas:
1 Medical services: ML is changing medical care by empowering early illness location, customized therapies, and medication disclosure. AI calculations can examine clinical pictures, anticipate patient results, and assist specialists with pursuing informed choices. For instance, ML-controlled models can recognize early indications of malignant growth in radiology examines or anticipate patient decay in light of fundamental sign information.
2 Finance: In finance, ML is utilized for algorithmic exchanging, extortion discovery, and hazard the board. ML models examine authentic market information to distinguish exchanging designs and anticipate stock costs. Essentially, credit scoring models use exchange information to evaluate the probability of advance reimbursement, diminishing the gamble for monetary foundations.
3 Internet business: AI drives proposal frameworks, which recommend items in view of a client’s perusing history and buying conduct. It’s additionally used to advance stock administration, estimating methodologies, and client support through chatbots and prescient investigation.
4 Independent Vehicles: ML is significant in the advancement of self-driving vehicles. These vehicles depend on calculations to decipher sensor information (e.g., cameras, radar, lidar) and pursue continuous driving choices, like route, deterrent aversion, and speed guideline.
5 Fabricating: AI further develops producing productivity through prescient upkeep. By examining information from sensors in machines, ML models can foresee when a machine is probably going to fizzle, considering opportune support and lessening personal time.
6 Regular Language Handling (NLP): ML is fundamental to NLP applications like discourse acknowledgment, language interpretation, and chatbots. Through procedures like profound learning, machines can process and comprehend human language, working with assignments like opinion examination, text rundown, and in any event, producing human-like text.
The Difficulties of AI
In spite of its immense potential, AI innovation faces a few difficulties:
1 Information Quality: The adequacy of a ML model is straightforwardly attached to the nature of the information. Deficient, one-sided, or boisterous information can bring about mistaken or unreasonable expectations.
2 Interpretability: Numerous ML models, particularly profound learning models, work as “secret elements.” It very well may be challenging to comprehend how a model showed up at a specific choice, which is tricky in enterprises like medical care or money where straightforwardness is basic.
3 Computational Expenses: Preparing complex models, particularly profound learning models, requires huge computational power and assets, which may not be available to all associations.
4 Moral Worries: AI can sustain inclination assuming it is prepared on one-sided information, prompting uncalled for or prejudicial results. Guaranteeing decency, straightforwardness, and responsibility in ML models is a developing area of examination.
The Eventual fate of AI
The fate of AI is unquestionably encouraging, with consistent progressions in calculations, information accessibility, and registering power. Regions, for example, support learning, logical computer based intelligence (XAI), and combined learning are pushing the limits of what ML can do. We can anticipate that ML should assume a much bigger part in independent frameworks, customized medication, and, surprisingly, imaginative ventures like workmanship and music.
Question and answer session on AI
Q1: What is the contrast among computer based intelligence and AI?
Computer based intelligence is the more extensive idea of making machines equipped for performing errands that ordinarily require human knowledge, for example, thinking, critical thinking, and learning. AI, a subset of man-made intelligence, explicitly centers around calculations that permit PCs to gain from information and further develop execution after some time without express programming.
Q2: Can AI work on over the long haul?
Indeed, AI models work on after some time as they are presented to additional information. Therefore consistent preparation and refreshing of models are vital to keeping up with high precision and execution.
Q3: How truly does AI affect occupations?
While AI can computerize specific assignments and diminish the requirement for difficult work, it additionally sets out new open doors. There is a rising interest for gifted experts in information science, AI designing, and artificial intelligence morals.
Q4: What are a few moral worries encompassing AI?
Key moral worries remember predisposition for preparing information, absence of straightforwardness in navigation (black-box models), and the potential for work relocation. Guaranteeing reasonableness, responsibility, and straightforwardness in ML frameworks is fundamental for moral artificial intelligence organization.
End
AI is an energizing, quickly developing innovation with the possibility to reform each industry it contacts. As AI keeps on propelling, its incorporation into day to day existence will just develop, making efficiencies, empowering advancement, and opening additional opportunities. Notwithstanding, to completely understand its advantages, it is urgent to address the difficulties of information quality, model interpretability, and moral ramifications, guaranteeing that the innovation is utilized mindfully and for everyone’s best interests.